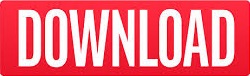
With respect to prevention of type I error it is still better to choose a method which under-estimates the effect ( conservative approach) than a method which might over-estimate it. Therefore, in clinical trials any over-estimation of the effect needs to be avoided. Or in other words: you increase the probability of a type I error. if you over-estimate the effect, you receive more likely a positive result. However, if you choose a too optimistic method of analysis, i.e.
According to the terminal drop hypothesis trial#
Thus, a high treatment effect leads to a successful trial (i.e. This is equivalent to a proof that “treatment A is actually better than treatment B” (that is the way statistical tests work). The aim is to disprove that “treatment A is not better than treatment B (so-called “ null hypothesis”). In a clinical trial (we only talk about superiority trials here as the situation is different for non-inferiority trials), one wants to detect a benefit of treatment A (e.g.

Thus, the common rule for clinical trial analyses is: be conservative! While “conservative” means: do not increase the probability of a type I error! Consequently, it is essential to keep the probability of situation A below the level of significance (e.g. 20%).Ĭoncluding, it is more essential to avoid a wrong proof than to avoid a wrong non-decision (which is also bad, but A is worse…). Situation B (known as type II error) on the other hand, is controlled via a meaningful sample size calculation, but usually with a less strict criterion (e.g. says that (if there is actually no effect) the probability of situation A is only 5% or less. Therefore, in clinical trials situation A (also known as type I error) is strictly controlled via a low pre-defined level of significance: a level of 5% e.g. However, the non-proven efficacy does not equal a proven inefficacy! From a scientific perspective, such a non-decision has less implications than a wrong proof. Situation B on the other hand means that efficacy was not proven but also not refused. Based on such a proof, an inefficacious treatment might be approved and patients put into danger. To answer this, consider the essential difference between the two cases:Ĭase A means that a statistically proven result is actually wrong – a result that might cause dangerous effects.
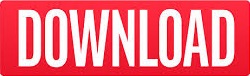